THE DEEP MODEL
Levels of Agency
When it comes to setting goals and thinking about how to achieve them, we all have our unique approaches, which can be very abstract or very concrete. Think about how you set goals in your life. Do you often think about big abstract questions like "What makes a happy life?" or "What's my long-term career plan?" These are abstract goals. They're broad, long-term, and often require many actions to achieve. On the other hand, some of us focus more on concrete, day-to-day objectives. Questions such as "What do I need to achieve today?" or "How can I improve my skills in this task?" are examples of concrete goals. These goals are more immediate, tangible, and often involve direct actions. In reality, everyone's mind wanders somewhere along this spectrum, from the abstract to the concrete, in the way we represent our goals and think about achieving them.
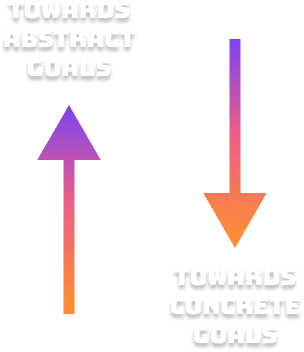
Exploration-Exploitation
Now, let's look at how we approach achieving these goals, whether they are concrete or abstract. Do you like to explore new ideas, try different things and embrace the unknown? That’s exploration – seeking out new experiences, learning new skills or finding new ways of solving problems. Conversely, do you prefer sticking to what you know, hone your existing skills and exploit familiar strategies? This is exploitation – optimizing what you already have and making the most of your existing knowledge and skills. In life, as in games, we constantly strike a balance between exploring new possibilities and exploiting known strategies.
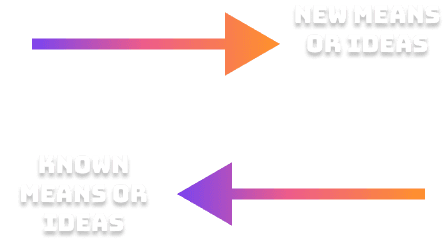
The four DEEP dimensions
The DEEP model offers a nuanced view of gaming preferences, where players aren't pigeonholed into a single category. Instead, each gamer has a unique profile on four dimensions, defined according to the two spectrums we discuss above: the concrete-abstract spectrum, and the exploration-exploitation spectrum. Each individual can have higher or lower scores on each of these 4 dimensions. This diversity reflects the multifaceted nature of gaming experiences. With this in mind, let's dive into each dimension:
Discovering: This dimension measures a player's interest in exploring abstract concepts in a game. Gamers who score high on this dimension are drawn to games that offer rich and complex worlds to unravel, such as the intricate lore of The Elder Scrolls series or the boundless universe of No Man's Sky. Lower scores may indicate a preference for more straightforward, action-oriented gameplay.
Expanding: Gamers who score high on this dimension are attracted to games with rich narratives and thematic depth. They find games like Mass Effect or The Witcher series captivating, where immersion in the story is key. Gamers who score lower on the Expanding dimension prefer games that place less emphasis on narrative and more on immediate gameplay mechanics.
Experimenting: Gamers who score high in Experimenting enjoy hands-on experimentation and learning through direct interaction. They thrive in games like Minecraft or Kerbal Space Program, where trial and error lead to tangible outcomes. A lower score in this dimension may suggest a preference for games with more defined rules or more structured gameplay.
Performing: Gamers who score high in Performing enjoy and excel at games where skill, precision, and efficiency are essential. Titles like Dark Souls and competitive esports games like League of Legends are typical favorites. A lower score on this dimension may indicate a preference for games that focus less on mastery and more on exploration or storytelling.
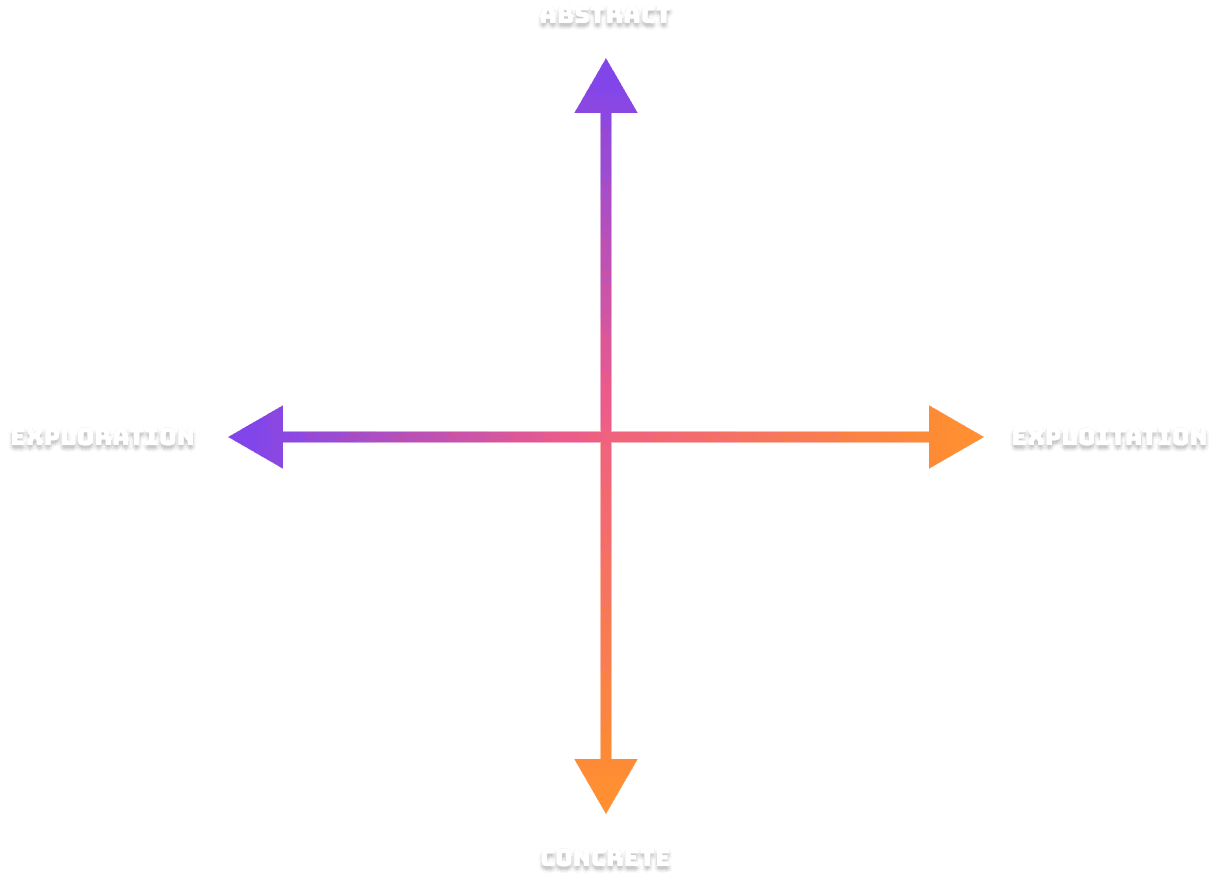
Psychometric scales
To determine these dimensions in the DEEP model, we used the factor analysis method. This process is akin to solving a complex, multi-dimensional puzzle. Imagine the individual preferences and behaviors of each player as puzzle pieces. At first, these pieces are jumbled together – it's not clear how they fit into the bigger picture. Factor analysis involves sorting these pieces into groups that naturally fit together. For example, all the preferences linked to the exploration of game worlds may cluster together, forming the 'Discovering' dimension of the puzzle.
An important step in this process was to check that these dimensions were consistent over time. Why is this important? Because if people's responses to these preferences change significantly over time, it could mean our 'puzzle' is not being put together correctly. We want to make sure that when someone gets a high score in, say, ‘Experimenting’, it really does reflect their gaming preferences, not just a passing mood or random choice. To test this, we asked gamers to fill in the questionnaire at different points in time and we checked the consistency of their responses. The consistency of score over time indicates that our dimensions are reliable and accurately reflect the stability of gaming preferences.
The predictive power of the DEEP model
To truly understand the value of the DEEP model, we needed to answer a key question:
Can the model predict the types of games a player would enjoy ?
First, we gathered data from 1,000 gamers who completed the DEEP questionnaire, which assessed their preferences along the four dimensions. We then asked them to list their 10 favorite video games. The challenge was to connect these two sets of data : the gamers' DEEP scores and their favorite games.
To bridge this gap, we turned to GPT, a large language model. GPT was trained on a huge amount of text, including vast amounts of gaming-related content from websites, forums and reviews. It's as if GPT has 'played' these games through the experiences and descriptions of countless gamers. We used GPT to annotate each video game on the four DEEP dimensions. The advantage of using GPT is its ability to provide consistent and comparable ratings across a wide range of games. This consistency is crucial as it allows us to compare apples to apples, so to speak, when correlating gamers' preferences with game characteristics.
After collecting the DEEP scores of gamers and their favorite games, we performed a correlation analysis to see how these two sets of scores relate to each other. Think of this analysis as drawing a line on a graph that best fits a scatter of points, where each point represents a gamer. One axis of the graph represents the gamer's DEEP scores and the other the DEEP scores of their favorite games. If gamers who score high on a particular DEEP dimension (such as Expanding) tend to favor games that also score high on that dimension, the points will cluster along the line in a pattern. This pattern – the correlation – is what we were looking for. A strong clustering of points along the line indicates a significant relationship, which shows that our DEEP model can predict with some effectiveness which games players will enjoy based on their individual gaming styles.
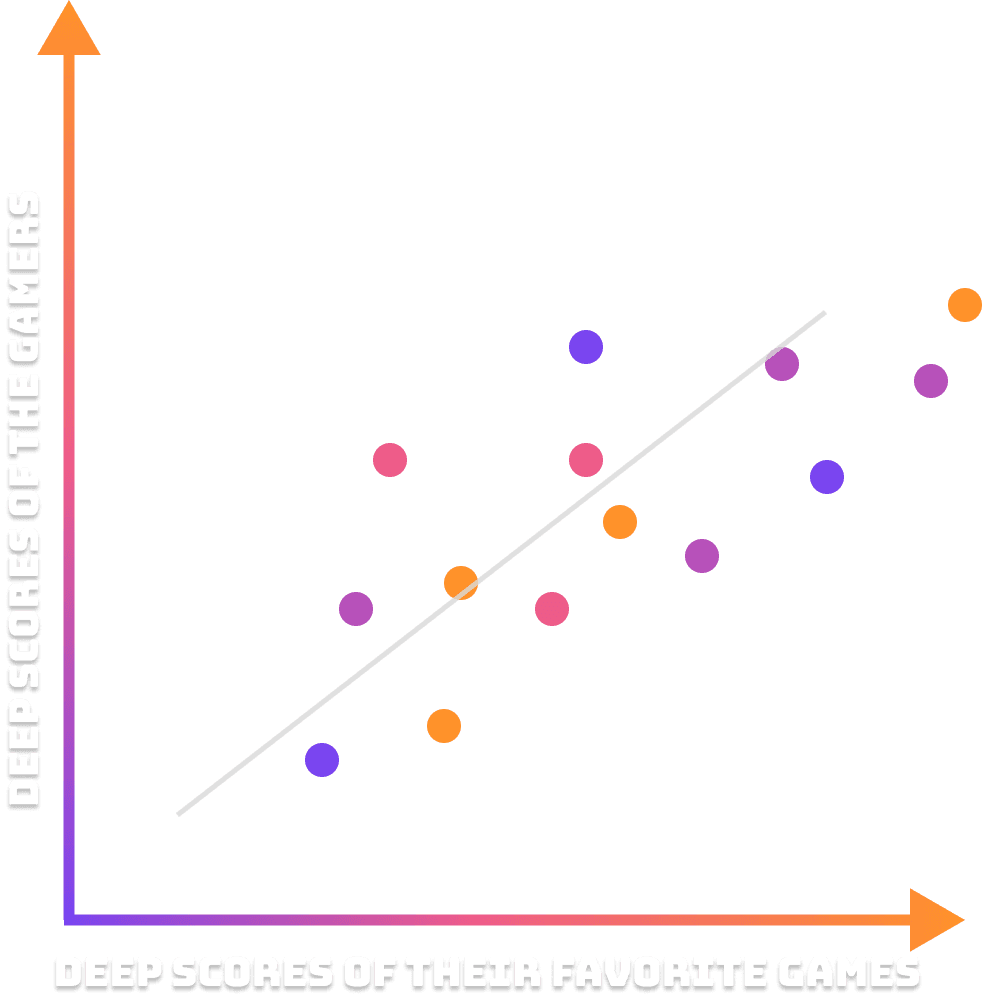